Databricks Data Analyzer
In the data analyzer stage, you perform analysis of the complete dataset based on selected constraints. For this you must add the Data Analyzer node to the data quality stage and then create a data analyzer job.
-
In the data quality stage, add a Data Analyzer node. Connect the node to and from the data lake.
-
Click the data analyzer node and then click Create Job to create the data analyzer job.
-
Provide the following information to create the Data Analyzer job:
Job Name
-
Template - this is automatically selected depending on the stages selected.
-
Job Name - Provide a name for the data analyzer job.
-
Node rerun Attempts - Specify the number of times the job is rerun in case of failure. The default setting is done at the pipeline level.
Click Next.
Source
-
Source - This is automatically selected depending on the type of source added in the pipeline.
-
Datastore - This is automatically selected depending on the configured datastore.
-
Source Format - Select Parquet or Delta table.
-
Choose Base Path - Click Add Base Path and select the path where the source file is located and then click Select.
-
Constraint - Select the constraint.
-
Column - Select the column and click Add.
Add the required constraints and click Next.
Target
-
Target - This is automatically selected depending on the type of target you select in the pipeline.
-
Datastore - This is automatically selected depending on the configured datastores to which you have access.
-
Choose Target Format - Select one of the following options:
-
Parquet
-
Delta Table
-
-
Target Folder - Select the target folder when you want to store the data analyzer job output.
-
Target Path - Provide an additional folder path. This is appended to the target folder.
-
Audit Tables Path - this path is formed based on the folders selected. This is appended with a folder Data_Analyzer_Job_audit_table.
-
Final File Path - the final path is created as /S3 bucket name/Target Folder/Target Path.
Click Next.
Cluster Configuration
You can select an all-purpose cluster or a job cluster to run the configured job. Since you are creating a custom transformation job, you may require certain library versions for successfully running the transformation job. To update the library versions, see Updating Cluster Libraries for Databricks.
In case your Databricks cluster is not created through the Lazsa Platform and you want to update custom environment variables, refer to the following:
All Purpose Clusters
Cluster - Select an all-purpose cluster from the dropdown list, that you want to use for the data transformation job.
Job Cluster
Cluster Details Choose Cluster Provide a name for the job cluster that you want to create. Job Configuration Name Provide a name for the job cluster configuration. Databricks Runtime Version Select the appropriate Databricks version. Worker Type Select the worker type for the job cluster. Workers Enter the number of workers to be used for running the job in the job cluster.
You can either have a fixed number of workers or you can choose autoscaling.
Enable Autoscaling Autoscaling helps in scaling up or down the number of workers within the range specified by you. This helps in reallocating workers to a job during its compute-intensive phase. Once the compute requirement reduces the excess number of workers are removed. This helps control your resource costs. Cloud Infrastructure Details First on Demand Lets you pay for the compute capacity by the second. Availability Select from the following options:
-
Spot
-
On-demand
-
Spot with fallback
Zone Select a zone from the available options. Instance Profile ARN Provide an instance profile ARN that can access the target S3 bucket. EBS Volume Type The type of EBS volume that is launched with this cluster. EBS Volume Count The number of volumes launched for each instance of the cluster. EBS Volume Size The size of the EBS volume to be used for the cluster. Additional Details Spark Config To fine tune Spark jobs, provide custom Spark configuration properties in key value pairs. Environment Variables Configure custom environment variables that you can use in init scripts. Logging Path (DBFS Only) Provide the logging path to deliver the logs for the Spark jobs. Init Scripts Provide the init or initialization scripts that run during the start up of each cluster. -
-
The Data Analyzer job is created. Click Start to run the data analyzer job. Alternately publish the pipeline and then run it to run the data analyzer job.
-
Once the job is complete, click the Analyzer Result tab. Click View Analyzer Results.
-
Depending on the selected constraints, you can view the results.
Note:
If you selected data type constraint in the data analyzer job, you see additional entries generated in the output results. See Data type constraints in data analyzer jobs.
You can download the results in the form of a CSV file.
-
Once the data analyzer job is complete and the results are available, the next step is to create a data validator job.
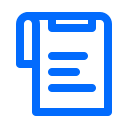
Note: The pipeline must be in Edit mode to create a data validator job.
Create a data validator job
-
Click the Data Analyzer node in the pipeline. First click the ellipsis (...) and then click Configuration.
-
Notice that the job now has an additional step of Validators added to it.
-
Provide the following information to create a data validator job:
-
Job Name
-
Template - this is automatically selected depending on the selected stages.
-
Job Name - provide a name for the data validator job.
-
Node Rerun Attempts - the number of times the job is rerun in case of failure. The default setting is done at the pipeline level.
-
Click Next.
-
Source
-
Source - this is automatically selected depending on the type of source added in the pipeline.
-
Datastore - this is automatically selected depending on the configured datastore.
-
Source Format - select either Parquet or Delta table.
-
Choose Base Path - this is automatically populated from the data analyzer path.
-
Constraint - the list of constraints selected in the data analyzer job is automatically populated. You can add additional constraints in the Validators step.
-
-
Validators
-
Do you want the pipeline run to be aborted if the validator result fails? - Enable this option depending on your requirement. If you enable this option, the pipeline run is terminated, if validator job fails.
-
Do you want constraints used in Data Analyzer to be used in Data Validator? – Click Add Constraints. Do one of the following:
-
Add New Constraints - Click this option to add new constraints. Select a constraint from the dropdown list. Select a column. Click Add. Repeat the steps to add all the required constraints. Then click Done.
Refer to Data Quality Constraints
-
From Data Analyzer - Click this option to view the list of constraints added in the data analyzer. Review the list and select a condition for the constraint, then click Add for the constraints that you want to add. Click Done once you have added the required constraints.
-
-
View the list of constraints that are added for the data validator job and then click Next.
-
-
-
Target
-
Target - this is automatically selected depending on the configured datastores to which you have access.
-
Choose Target Format - select either Parquet or Delta table.
-
Target Folder - select the target folder where you want to store the data validator job output.
-
Target Path - you can provide an additional folder path. This is appended to the target folder.
-
Audit Tables Path - this path is formed based on the folders selected. A folder Data_Analyzer_Job_audit_table is created for data analyzer and another folder Data_Analyzer_Job_audit_table_validator is created for data validator.
-
Final File Path - the final path is created as follows: /S3 bucket name/Target Folder/Target Path
-
-
Cluster Configuration
You can select an all-purpose cluster or a job cluster to run the configured job. In case your Databricks cluster is not created through the Lazsa Platform and you want to update custom environment variables, refer to the following:
-
Select an All Purpose Cluster - this is already configured. Select one from the dropdown.
-
Job Cluster - Provide the required details to create a job cluster.
Click Complete.
-
-
Click the data analyzer node and click Start to initiate the data validator job run.
-
Once the job is successful, click the Validator Result tab. Click View Validator Results.
What's next? Databricks Issue Resolver |